Reimagining the Lookalike for Digital Acquisition
9/3/2024 Jake Hall
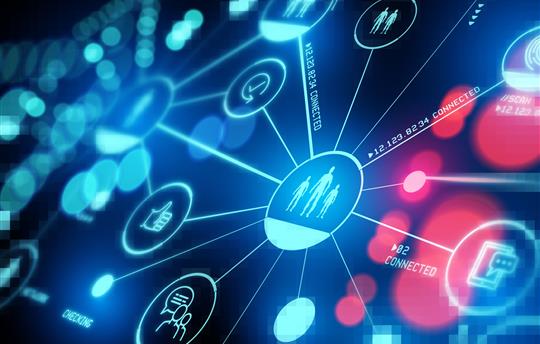
What does it mean to "look alike"?
How would you describe someone who’s “just like you”? I am, for example, a 45–54-year-old male with a wife and 2-4 children living in a single-family home in a semi-rural area of Upstate New York. My neighbor is too — are we alike? Of course, the answer depends on context and invariably leads to something reductive.
We instinctively know this: we have friends, belong to communities and organizations, and live and work in different circles defined by often very different characteristics and commonalities. So, why do we think we can just upload a list of customers and receive an audience of lookalikes based on some generic algorithm? The easy answer is that it’s still better than random and that’s just how it’s done. But there is a smarter way.
Understanding customers through their relationship with a brand
The answer is simple — the best way to understand how to describe a group is by looking at what brings them together. When thinking about customers, we need to define what they look like in the context of their relationship with a brand. For some, the predefined lookalike model may happen to be a perfect fit. However, most brands would contend they have something unique that describes their ideal customers. The trick is not only figuring out how to define this uniqueness, but also figuring out how to make it actionable.
The data ecosystem: an intersection of insights
Our industry sits at the confluence of massive streams of data. DSPs live on the edge, observing a torrent of raw bidstream data and enabling a contextual but highly anonymized view of consumer interests and behavior. Brands sit on the other side, interacting with their customers directly both online and in person. Of course, demographers and data compilers live somewhere in the middle, looking at census and other data sources to construct a terrestrial view of households and the individuals within.
Ideally, we would start by looking through the lens of the brand and seeing what makes a good customer, who brings the greatest lifetime value, and how they are different from other customers and, more importantly, what I’ll call “never customers.” We can use this insight to predict the propensity for a prospect to become a customer for that brand, not just determine who “looks alike.”
The challenge of disparate data streams
For many of us, disparate data isn’t breaking news. The problem is that making use of these varied and unrelated data streams is challenging on multiple levels. While many of these challenges are technical, marketers cannot afford to lose sight of the important and evolving concerns around consumer preferences and privacy regulations.
Approach to customer data
Starting with a profound respect for customer data and its power to help brands build better relationships with consumers, the consumer data layer of a robust platform like RRD’s NXTDRIVE™ works like a CDP to securely house and organize first-party data. It also functions as a platform to host analytics and serves as a hub for the martech and adtech sides of the ecosystem to come together. The consumer data layer takes care of first-party identity management, providing data hygiene, customer identification, and householding. This is also where additional layers of first-party data are brought in to build the proverbial 360-degree view of customer activity — including transactional, web browse, and campaign response information.
Next, RRD’s Consumer Graph — covering 130 million U.S. terrestrial addresses, 110 billion daily intent signals, and 33 billion daily location signals — is used to create a bridge. This is where terrestrial and digital data are stitched together at varying levels of resolution to develop over 1,500 variables for data scientists to work their magic. Since the connection between online and offline data is imperfect, particularly when bridging first- and third-party data sets, machine learning and AI are leveraged to identify patterns. This helps impute missing data and rank relationships between different levels of identity and geography, enabling a consistent dataset on which to train a custom propensity model.
Tailoring models for maximum predictiveness
While much of the model training can be streamlined for efficiency, each model is hand-tuned by data scientists to ensure they’re as predictive as possible before they are installed into a high-performance production environment. This enables us to build a predictive model that can evaluate any prospect in our graph for their propensity to become a customer of your brand. The result? No more generic lookalikes.
Bridging online and offline acquisition opportunities
Instead of pushing first-party data to the advertising platform for lookalikes, our platforms ensure that ad tech is pulled into the CDP. This creates a CDMP, bridging online and offline acquisition opportunities and enabling more efficient media decisions and better outcomes aligned with your unique customers and goals.
So, I’m actually 47 and only have 2 kids, but if you’re selling home goods, you’d rather know that I, like many of your best customers, love cooking and DIY projects, and consume a lot of news and technology content. And, if you’re selling farm and fleet, my semi-rural home seven miles from your store is great news — but even better news is that I have chickens (and my neighbor does not).
Jake Hall is the Senior Director of Strategic Development, NXTDRIVE at RRD. For more information on harnessing the full potential of your first-party data, visit rrd.com/nxtdrive.